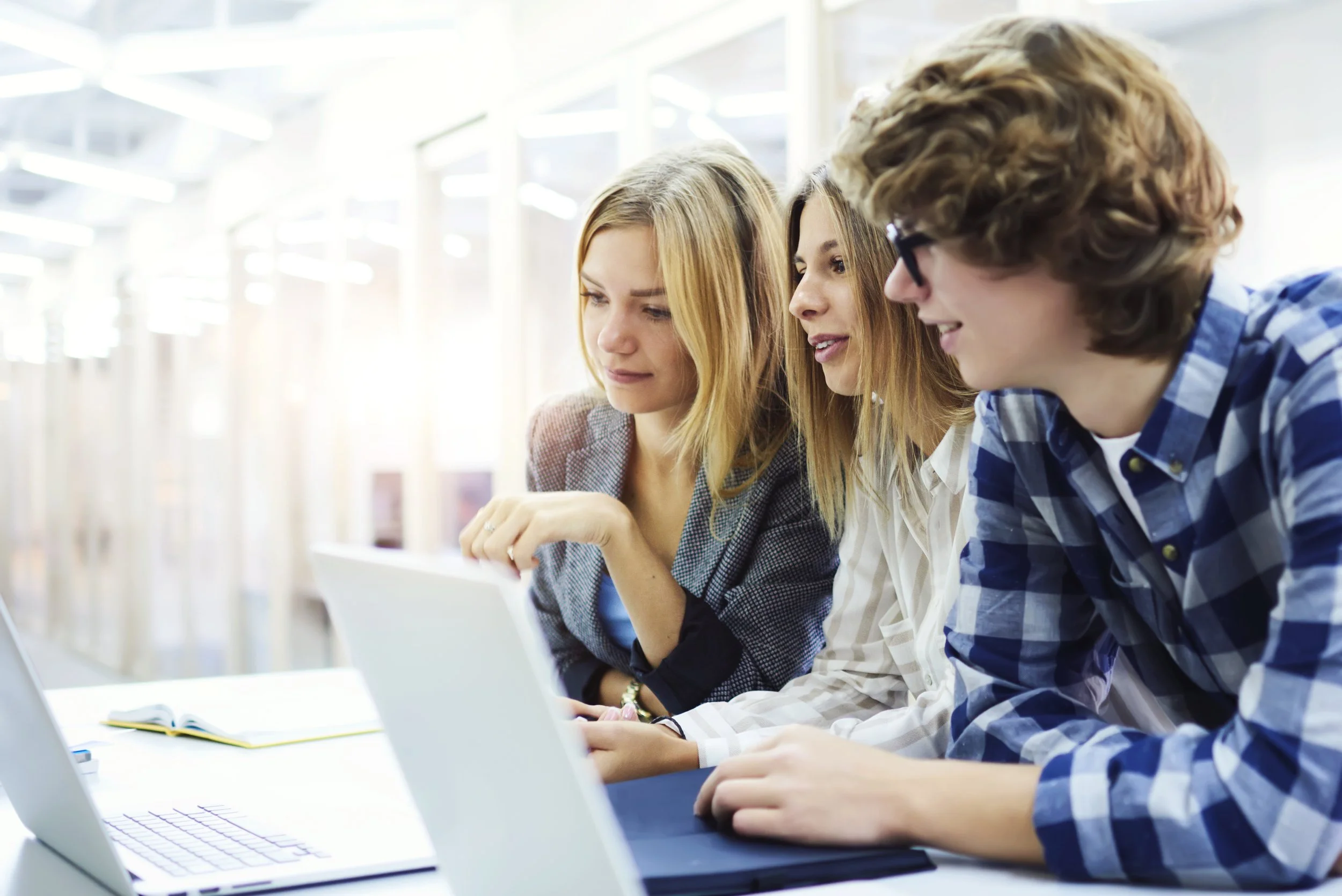
Internships
Ignota Labs are delighted to welcome interns to join our team. Our internships offer first-hand experience working in a TechBio start-up and provide the opportunity to deliver significant impact by contributing as part of our team. Individuals can choose a project that aligns with their skills and interests such as software development, machine learning, business development and drug discovery.
Over a flexible period, interns will gain insight into the start-up environment and gain personal insight into the role of AI and machine learning in the future of drug discovery. Additionally, given our small team, interns will get to work directly with co-founders to catch a glimpse of what it takes to found a company and inspire future entrepreneurs.
Hybrid working: Ignota Labs operates a hybrid policy with staff working between home and our offices in Cambridge science park. The team gets together for 1-2 days per week for whole-team collaboration days, and we use Gather Town and Slack to promote communication and team working when operating remotely. Remote only arrangements may be considered, although we encourage face-to-face collaborations whenever possible.
Duration and funding: We welcome PhD students for flexible durations as part of CDT or other programs with internship provisions. We can offer reasonable expenses to cover travel to our Cambridge offices for collaboration days. In exceptional circumstances, we will consider applications from masters students. Other paid internships are available in cycles, with our next wave coming in September 2024.
Application process: We welcome all applicants via email at info@ignotalabs.ai. In your email please include a copy of your CV as well as your preferred start date, internship duration and project preference. For candidate led project proposals, please include a short paragraph about your interests and how they align with Ignota Labs.
-
Introduction: Publicly available chemical toxicity datasets such as ChEMBL and PubChem contain a wealth of data, which can be used for machine learning-based toxicity prediction. However, these datasets come from a mixture of in vitro and in vivo assays that are not always comparable. As a result, the machine learning models trained on these datasets introduce noise, which affects their accuracy. Therefore, this project aims to develop an approach to contextualise chemical toxicity datasets based on the scientific understanding of the biological mechanisms involved in different types of toxicity. This project will involve specific activities and outcomes, as described below:
Activities:
1. Collecting and integrating chemical toxicity datasets: Publicly available chemical toxicity
datasets will be ingested from different sources such as ChEMBL and PubChem. These
datasets will be integrated into a unified dataset for analysis.
2. Data pre-processing and cleaning: Data will be cleaned and processed to remove any
inconsistencies and errors, and to standardise data format.
3. Biological mechanism-based data contextualisation: Scientific literature review to identify
the biological mechanisms involved in different types of toxicity and the assays used to measure them. Based on this understanding, the data will be cleaned and labelled according to the mechanism involved in toxicity and the comparability of assay types.
4. Machine learning model development: Machine Learning (ML) models to be developed for toxicity prediction using the contextualised dataset. The models will be evaluated using cross-validation and external validation datasets using Ignota Labs’ proprietary ATLAS
automated ML platform.
5. Analysis of model performance: Performance of the machine learning models to be evaluated and compared with the models trained on the original datasets.
Outcomes:
1. A unified and cleaned dataset of chemical toxicity data.
2. An approach for biological mechanism-based contextualisation of chemical toxicity
datasets.
3. Machine learning models for toxicity prediction, trained on contextualised data.
4. An evaluation of the performance of machine learning models trained on contextualised
data compared to the models trained on original datasets.
5. A research paper summarising the approach, methods, and findings of the project.
Impact: The proposed project aims to enhance the accuracy of machine learning models for toxicity prediction by contextualising chemical toxicity datasets based on biological mechanisms. This approach will reduce the noise in the data and provide a better understanding of the biological mechanisms involved in toxicity. The models developed in this project will have the potential to be applied in the pharmaceutical industry for drug discovery and development, and in the regulatory agencies for toxicity assessment. The approach developed in this project will be widely applicable to other areas of machine learning-based predictions where the data is heterogeneous and noisy. -
Introduction: Preclinical safety evaluation of new drug candidates is a crucial step in the drug discovery and development process. Currently, in vitro assays are used to evaluate toxicity, but their predictive power in humans or pre-clinical species is limited. Therefore, there is a need for in vivo relevant toxicity models that can better predict the safety of drug candidates in humans. This project aims to develop in vivo relevant toxicity models using knowledge graphs, which integrate diverse biological data sources to provide a more comprehensive understanding of toxicity mechanisms. The project will involve the following activities and outcomes:
Activities:
● Data will be collected from diverse biological data sources, including genomics,
proteomics, metabolomics, and transcriptomics data, to develop a comprehensive knowledge graph.
● A knowledge graph-based framework will be developed that integrates the collected data
and facilitates the identification of relevant biological mechanisms for toxicity prediction.
● Relevant in vivo data from animal studies and human clinical trials will be annotated in the
knowledge graph to identify the most relevant mechanisms for toxicity prediction.
● Machine learning models will be developed using the annotated knowledge graph to
predict toxicity. The models will be evaluated using cross-validation and external validation datasets.
● The performance of the machine learning models will be analysed in terms of both accuracy and ability to recapitulate molecular mechanisms of toxicity (Explainable AI)
Outcomes:
The proposed project aims to enhance the accuracy of machine learning models for:
● A comprehensive knowledge graph integrating diverse biological data sources will be created.
● A knowledge graph-based framework for in vivo relevant toxicity prediction will be developed.
● Machine learning models for toxicity prediction, trained on annotated knowledge graphs, will be created.
● An evaluation of the performance of machine learning models trained on annotated knowledge graphs compared to the models trained on original datasets will be conducted.
● A research paper summarising the approach, methods, and findings of the project will be written.
Impact: The project aims to develop in vivo relevant toxicity models using knowledge graphs which will enable more accurate and predictive safety evaluation of drug candidates. The models developed in this project will have the potential to reduce the time and cost of drug development by providing better preclinical safety evaluation. The knowledge graph-based framework developed in this project will also be widely applicable to other areas of biomedical research where integrating diverse data sources can provide a more comprehensive understanding of complex biological processes.
-
Introduction: The development of accurate and reliable toxicity prediction models is crucial in drug discovery and development. Currently, several machine learning architectures and molecular representations have been employed in toxicity prediction models. However, there is a need to develop novel architectures and representations to improve the accuracy and robustness of these models. This project aims to develop and benchmark novel architectures and representations for toxicity prediction against current approaches. The project will involve specific activities and outcomes, as described below:
Activities:
● A review of current machine learning architectures and molecular representations used in toxicity prediction models.
● The development and implementation of novel architectures and representations, such as graph neural networks and attention mechanisms.
● The training of the novel architectures and representations and current approaches using publicly available toxicity datasets.
● The benchmarking of the novel architectures and representations against current
approaches using different metrics, including accuracy, sensitivity, specificity, and area under the curve (AUC) of the receiver operating characteristic (ROC) curve.
● A thorough analysis of the performance of the novel architectures and representations
compared to current approaches.
● Identification of the most promising novel architectures and representations.
Outcomes:
● A review of the current machine learning architectures and molecular representations used in toxicity prediction models.
● The project aims to develop in vivo relevant toxicity models using knowledge graphs,
● The development and implementation of novel architectures and representations for toxicity prediction.
● A comprehensive benchmarking of the novel architectures and representations against current approaches.
● Identification of the most promising novel architectures and representations for toxicity prediction.
● A research paper summarising the approach, methods, and findings of the project.
Impact: The proposed project aims to develop and benchmark novel architectures and representations for toxicity prediction. The project will contribute to improving the accuracy and robustness of toxicity prediction models, leading to better preclinical safety evaluation of drug candidates. The identification of promising novel architectures and representations will also pave the way for further research in this area, leading to the development of more effective models for toxicity prediction. The research findings and the new models can be widely applicable to other areas of chemical biology research.
-
Introduction: Omics data plays a fundamental role in elucidating various biological processes and in understanding the intricate mechanisms underlying different conditions.
However, extracting omics data can be a challenging and time-consuming task, involving various steps of manual curation and metadata analysis. Language Models (LMs) offer a promising avenue to streamline this process, automating the extraction of relevant data from open-source publications.
Activities:
Identify the most suitable Language Model model API (BERT, Perplexity, GPT, etc)
Determine the type of omics data required (genomics, metabolomics, etc) and the level of preprocessing more suitable to our applications (raw, preprocessed, etc)
Identify the source/s of data (PubMed, bioRxiv, GenBank, etc) and the preferred output
Handle scraping (pagination, server blocking scraping, etc)
Identify a way to test the ground truth (it could be by providing manual examples to test that the model is correctly retrieving relevant omics data)
Precision and Recall can be tested as suggested by this paper (https://www.nature.com/articles/s41467-024-45914-8#Sec8)
Develop a simple interface
Outcomes:
Delivery of a machine learning tool capable of aggregating datasets from diverse sources, empowering subsequent ML model development and analysis
A research paper summarising the approach, methods, and findings of the project
Development of valuable engineering and research skills among team members, fostering growth and expertise in cutting-edge technologies
Impact: The project aims to develop a model capable of identifying data and aggregating it from different sources offering a solution to a traditionally manual and time-consuming process.
-
Introduction: Reducing redundancy in pathway enrichment analysis results is critical for improving the interpretability of biological data and to focus on the most relevant pathways. By unifying similar pathways and reducing the influence of overinflated pathways (like cancer), researchers can uncover novel biological insights. This, in turn, contributes to a clearer understanding of the underlying biological processes driving specific phenotypes or conditions.
Activities:
Decide on the input for the model (list of pathways, datasets, p-vals, etc)
Preprocess data (remove duplicates, not relevant processes)
Identify the most suitable pre-trained Language Model model (GPT-3, or others, LLAMA2) - we are looking for semantic similarity here
Device a way to keep into account semantic similarity and biological relevance (some sort of hierarchical structure would be helpful but also considering p-values) - this could be done via clustering similar pathways together
Assign representative pathway names or descriptions to each cluster based on the pathways it contains
Evaluate with ground truth manual examples (or thinking of a way to measure redundancy)
Might also need input from a scientists
Outcomes:
Delivery of a machine learning tool capable of processing enrichment analysis result
A research paper summarising the approach, methods, and findings of the project
Development of valuable engineering and research skills among team members, fostering growth and expertise in cutting-edge technologies
Impact: By using language models, pathways are harmonised, reducing the impact of overrepresented entries such as “cancer”. These results enable a better understanding of biological processes and clearer results.
-
Introduction: Testing the effects of different drugs at different doses in various cell lines can be costly and time-consuming. However, predicting the effects of drug perturbations across different cell lines and at different doses could be a helpful way to expand our knowledge on the effects of a given compound on the body.
This project would involve the adaptation/improvement of the paper “Generative modelling of single-cell gene expression for dose-dependent chemical perturbations [1]” and the construction of a UI to make it easy to generate and inspect the data.
[1] https://doi.org/10.1016/j.patter.2023.100817
Activities:
Identify the right architecture/paper to start with
Start by reproducing the results of the model
Explore a toy example to make sure the results are as expected
Identify problems and suggest improvements with the current architecture
Build a simple UI
Outcomes:
Delivery of a machine learning model capable of predicting the effects of drug perturbations in different cell lines
A research paper summarising the approach, methods, and findings of the project
Development of valuable engineering and research skills among team members, fostering growth and expertise in cutting-edge technologies
Impact: By using generative models, we can predict (in-silico) the effects of new small molecules on cell lines that have not been previously tested. This can be useful to model the effects of drugs and for hypotheses generation.
-
There is an opportunity for interested students to work with us to develop an alternative project to those listed that best reflects their skills and interests. Ignota Labs are excited to engage with and foster emerging talent and welcome applications from a range of students.
“Interning at Ignota Labs has shown me the dynamic nature of working in AI and has constantly challenged me to adapt and learn new skills, making this internship an invaluable experience”
Lianne G, Toxicology Intern